A predictive machine learning model for Alzheimer’s disease
The study aims to develop a screening algorithm for the identification of the Alzheimer’s disease (AD) conversion in subjects with MCI, based only on easily collectable, non-invasive and inexpensive predictors.
Abstract
Despite the increasing availability in brain health related data, effective methods to predict the conversion from Mild Cognitive Impairment (MCI) to Alzheimer’s disease (AD) are still lacking. As currently available and emerging therapies have the greatest impact when provided at the earliest disease stage, the prompt identification of subjects at high risk (e.g. MCI patients) for conversion to full AD is of great importance in the fight against Alzheimer’s disease. In the current era of big data, advanced computation over large amounts of brain images, electronic health records, and wearable devices may enable new insights into the working mechanisms of the human brain. A predictive machine learning algorithm based only on non-invasively and easily collectable predictors potentially allows us to identify MCI subjects at risk for conversion to full AD. Although these new technologies also reveal potential challenges and ethical questions, an applicable screening algorithm would ensure better selection of subjects to include in clinical trials for preventative treatments, and allows early identification of subjects who would benefit the most from such treatments.
Project Team
- Nadine Rouleaux
- Massimiliano Grassi
- Michel Dumontier
- Technical/infrastructure support: Alexander Malic, Pedro Hernandez Serrano, Seun Adekunle
Poster
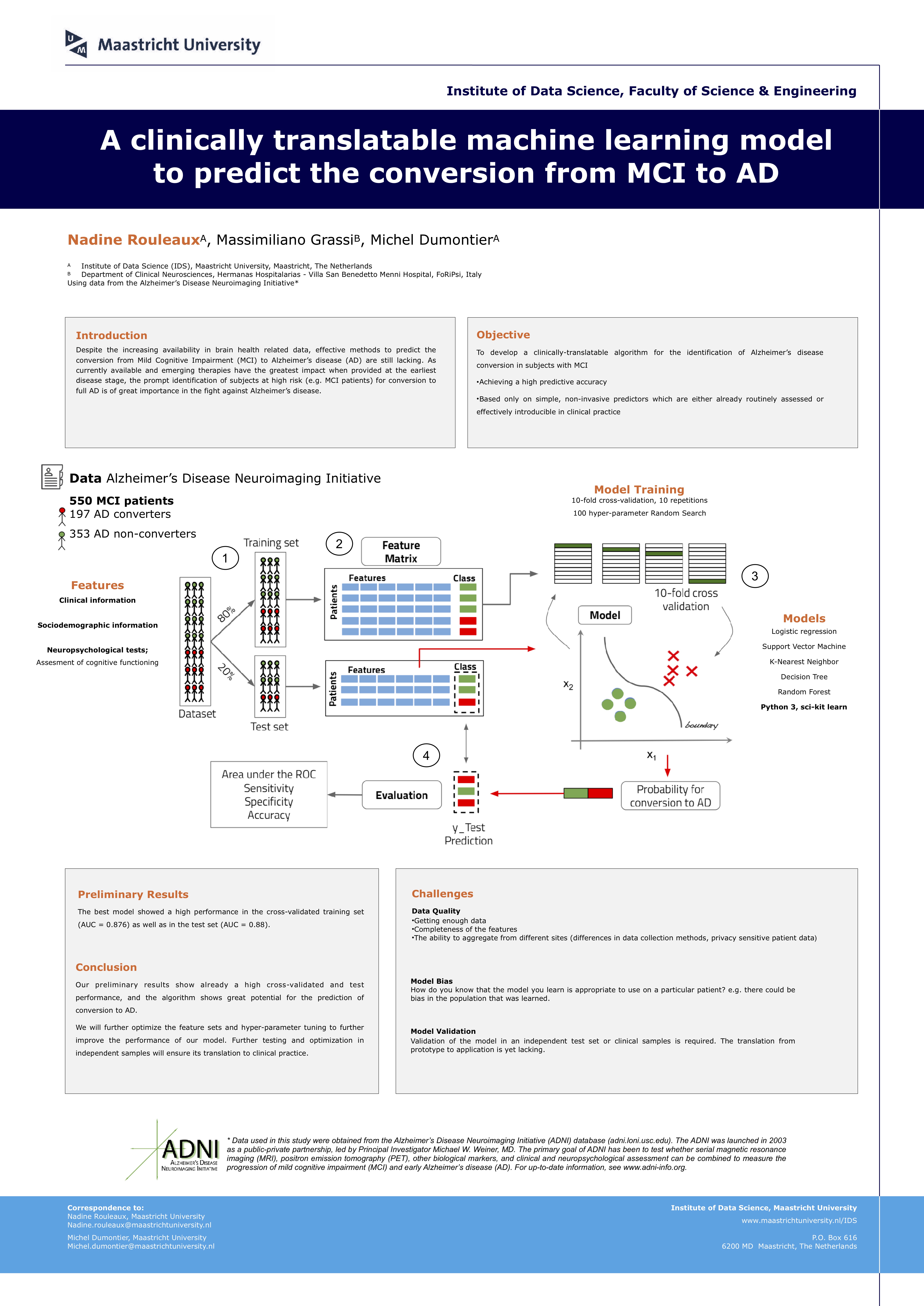