Smart devices tailor their teaching to your emotions
For the European Horizon 2020 innovation project MaTHiSiS, researchers taught machines how to teach you by learning from watching you learn. Wait, did that go too fast? Apologies - tailoring a learning curve is no easy feat for a human being, let alone for a machine.
Let’s try again, starting from a more simple learning experience: The color of a ripe tomato is red.
So, how did that make you feel? Perhaps you enjoyed learning something new. Maybe you were offended because you already knew, or you experienced one of the many other possible emotions upon being presented with this neat little fact.
But I don’t like learning
What if your computer, your tablet, or even a robot recognised how you felt while learning about the color of tomatoes? And what if it used this kind of information to tailor your learning curve, so it could ensure the best possible learning experience for you? It may sound like science fiction, but such a vision underpinned the Horizon 2020 Innovation Action 'MaTHiSiS'. The project recently reached its conclusion following three years of work from 18 different partners across the European Union.

Department of Data Science and Knowledge Engineering
Red is more than just the colour of ripe tomatoes: MaTHiSiS is here seen running on a so-called TurtleBot, which follows a researcher around the room. The researcher was tasked was tasked with showing it five red objects. Meanwhile, MaTHiSiS keeps track of the researcher’s engagement and performance.
“Basically, we wanted to build a learning framework that optimizes the learning experience according to the emotions and performance of each individual learner. We were looking at all possible cues: your face, your posture, the way you speak, how well you were doing with the tasks. Furthermore, MaTHiSiS had to run on anything from classical PCs all the way up to robots”, says Dr. Stelios Asteriadis, associate professor at the Department of Data Science and Knowledge Engineering (DKE).
“Our job was to combine all those different cues, and to set up the automated recognition of facial expressions. We also enabled the use of artificial intelligence to map simple digital interactions to emotions and, at the same time, made sure all of this could be used to optimize the learning experience.”
Basically, we wanted to build a learning framework that optimizes the learning experience according to the emotions and performance of each individual learner.
Dr. Stelios Asteriadis
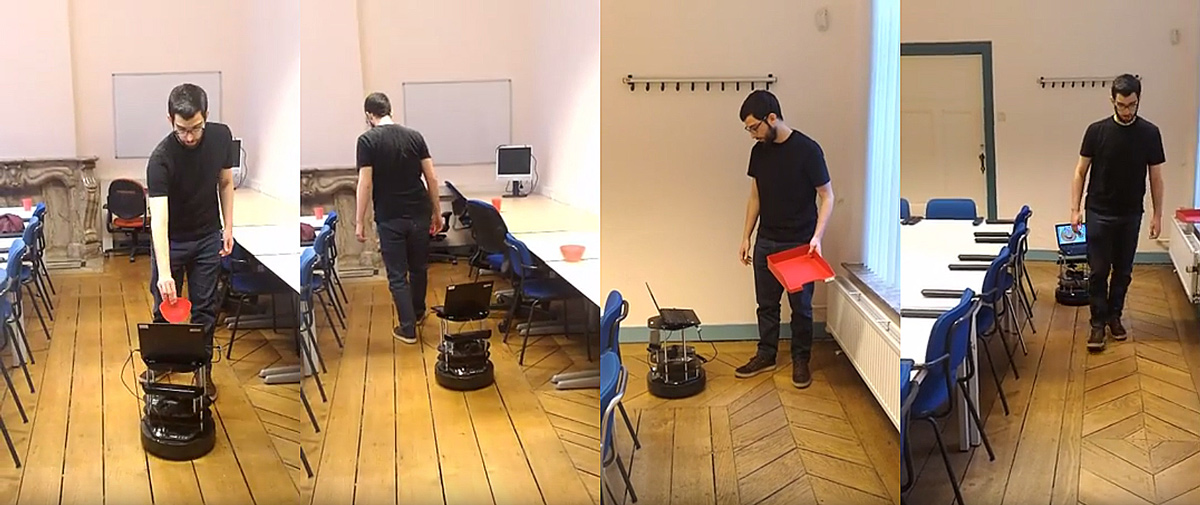
Why care about feelings during learning?Negative emotions such as frustration or stress can undermine one’s ability to learn. Similarly, people who feel engaged while learning perform better. Various cues (facial expression, tone of voice and posture, for example) reveal how someone feels at a specific moment. MaThiSiS’ algorithm analyses these cues and adjusts its teaching, so that the learner can perform optimally. Of course, successfully building a learning method incorporating emotions requires more than just technical know-how of algorithms and artificial intelligence. As such, the MaTHiSiS team also included pedagogists and psychologists. |
Interestingly, the project itself also turned into a learning experience for Dr. Asteriadis and his team. “When the project started, we knew that learning is based on emotion, and that positive emotions are good for learning. However, we didn’t see the pedagogical theories up close until meeting with the psychologists and pedagogists involved in the project. We need to work more closely with them from the beginning, because this collaboration worked very well in this project. It’s definitely something we will impose on ourselves in the future.” |
MaTHiSiS was funded by a Horizon 2020 research grant from the European Union. For more information: http://mathisis-project.eu/
An early version of MaTHiSiS’ artificial intelligence showing what emotions it perceives from the researcher’s facial expressions, in real time.
“One of the techniques we applied to solve this problem is so-called transfer learning.” In this case, the algorithm first learned to recognize emotions by finding patterns in data from the overall population. For example, it learned what happy people usually look like, what angry people usually look like, and so on. For this, the algorithm made use of existing, publicly available datasets. “During interaction between MaTHiSiS and the learners, we then kept improving the algorithm further. The end goal is to have the algorithm analyse your feelings and recommend what you need, at this specific moment.”
But I already know all of this!
A second challenge comes from the fact that people spend every waking moment learning. “Nowadays, we are surrounded by social media and all sorts of devices. We continuously learn in different ways, even if we are unaware of it.” Learning things you already know isn’t particularly engaging. With so many informal opportunities to learn, the level of prior knowledge varies wildly. This too calls for a personalised approach to learning.
“Suppose the big goal is to learn math”, says Dr. Asteriadis. “You need to learn addition, subtraction, division, and so on.” Teaching these concepts in a fixed order risks boredom in learners who are already familiar with some of them. Therefore, the team went with a non-linear way of teaching. “We broke learning material down into chunks of smaller learning subgoals, so that the algorithm could jump from one subgoal to another. The algorithm determined your learning path based on your performance and your emotions. The Maastricht team was responsible for this adaptation as well, for allowing the system to recommend the best material for a particular student in a particular moment.”
But… Wait, this kinda works
MaTHiSiS was extensively tested in five different countries across Europe, with a focus on students with special needs. “The results were very encouraging. Among other things, we looked at the engagement of learners compared to the emotion recognition we derived, and found that they were correlated.” Put simply, that means that the emotion recognition algorithms could successfully identify when someone was engaged in learning. “Pedagogists also compared learning outcomes with and without MaTHiSiS, and with and without emotion recognition. We are now exploring opportunities to refine, reuse and retest the system in different environments.”

The UM team working for MaTHiSiS. From left to right: Esam Ghaleb (PhD candidate), Matteo Amestoy (research assistant), Stelios Asteriadis (associate professor), Enrique Hortal (postdoctoral researcher) and Christos Athanasiadis (PhD candidate).
But today just feels, like, wrong for studying
In real life situations, recognising emotions isn’t as easy as it seems. “Don’t forget that different people express their emotions in different ways. It even changes within the same person: one day I may express my emotions in one way. The next day, I could be moody and I express my emotions in a different way. This is challenging to overcome for a machine.”
Don’t forget that different people express their emotions in different ways.
Also read
-
On 01 October, the Faculty of Science and Engineering opened its doors to host 'Weekend van de Wetenschap' (English: Weekend of Science).
-
Scientists from the Brightlands Institute for Smart Society (BISS) at Maastricht University are helping to find solutions to poverty and debt.
-
Simon Schick (27) a PhD Candidate at Aachen-Maastricht Institute for Biobased Materials (AMIBM). He lives together with his fiancé in Dusseldorf. Simon is currently working on the Marie Sklodowska-Curie BioBased Value Circle Project.