UM Data Science Research Seminar
The UM Data Science Research Seminar Series are monthly sessions organized by the Institute of Data Science, in collaboration with different departments across UM. The aim of these sessions is to bring together scientists from all over Maastricht University to discuss breakthroughs and research topics related to Data Science. This seminar is organized in collaboration with Precision Medicine.
N.B.: All events are in-person and free of charge. We also offer participants a FREE lunch.
Schedule
LECTURE 1
Time: 12:00 - 12:30
Speaker: Astrid van Camp
Title: "Creating synthetic data to overcome data scarcity in deep learning model training: an application to contrast-enhanced mammography"
Abstract: Training deep learning (DL) models to perform tasks such as detection or classification often requires large amounts of data. In medical imaging, this can be challenging due to a lack of available and annotated data. To overcome this data scarcity problem, datasets can be enriched with synthetic images.
Unlike digital mammography, which many countries apply in their screening program, contrast-enhanced mammography (CEM) is mostly used for recalled and high-risk breast cancer patients. This limited availability of CEM data presents difficulties for DL models in achieving high performance. Therefore we introduce models to generate synthetic CEM images with a focus on the breast cancer lesions that are least common or most difficult to detect. Next to a simulation pipeline, which considers the clinical properties of a breast lesion and models the physical acquisition, we also look into DL-based models such as generative adversarial networks. With the generated synthetic images, we can augment our training data and improve the detection of lesions in CEM data.
LECTURE 2
Time: 12:30 -13:00
Speaker: Zohaib Salahuddin
Title: "Counterfactuals and LRP interpretability framework for predicting symptomatic post-hepatectomy liver failure in patients with hepatocellular carcinoma”.
Abstract: Two-dimensional shear wave elastography (2D-SWE) has demonstrated predictive value for symptomatic post-hepatectomy liver failure (PHLF) in hepatocellular carcinoma (HCC). However, routine analysis of 2D-SWE images fails to take full advantage of all the image information. We enrolled 345 consecutive patients, including 265 patients in the training cohort and 80 patients in the test cohort. We used β-Variational Autoencoder to learn disentangled representations of the SWE image. A multi-layer perceptron (MLPswe.) model was trained using the latent representations of β-VAE for PHLF prediction. Clinical variables were also included along with the latent representation for training MLP models for PHLF prediction, denoted as MLPclinical-swe. We exploited the continuity in the latent space of β-VAE to perturb the latent space and generate counterfactuals for explainability. We used Layerwise relevance propagation (LRP) to investigate the contribution of SWE latent representations and clinical variables in MLPclinical-swe in terms of local and global explanations. During five-fold cross-validation, MLPclinical-swe, MLPswe, Densenet-121, and Resnet-18 models achieved an AUC of 0.849, 0.759, 0.745, and 0.703, respectively. In the test cohort, MLPclinical-swe model showed an AUC of 0.828, which was higher than the clinical model (AUC 0.684, p = 0.007), MELD score (AUC 0.529, p < 0.001), and ALBI score (AUC 0.644, p = 0.016). We proposed an interpretability framework based on counterfactuals and layerwise relevance propagation that is explainable and demonstrates superior performance compared to the clinical model.
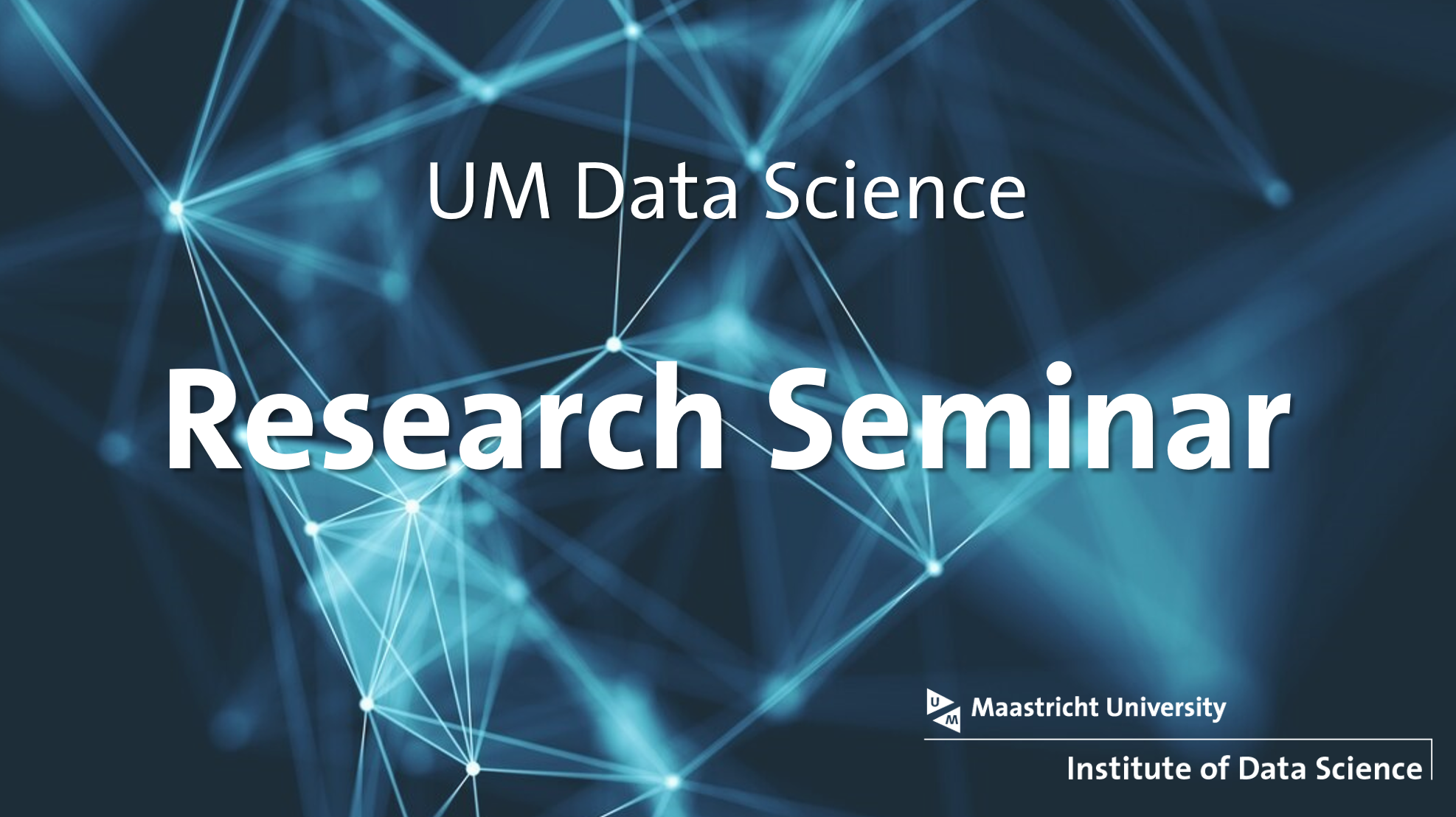
Please register by Thursday 13 April, 10:00 A.M. (CEST).
Also read
-
05 Aug16:00 - 17:00
Faculty of Health, Medicine and Life Sciences | Campus tour for prospective bachelor's and master's students
We offer the possibility of a guided campus tour. One of our students is happy to take the time and guide you around the campus, answering all the questions you might have.
-
20 Aug19:00