UM Data Science Research Seminar
The UM Data Science Research Seminar Series are monthly sessions organised by the Institute of Data Science, on behalf of the UM Data Science Community, in collaboration with different departments across UM with the aim to bring together data scientists from Maastricht University to discuss breakthroughs and research topics related to Data Science.
This session is organised in collaboration with Precision Medicine Maastricht (The D-Lab).
Schedule
Presentation 1
Time: 12:00 - 12:30
Title: Interpretability Methods for Mass Classification and Segmentation in Mammograms.
Speaker: Zohaib Salahuddin
Abstract: Artificial Intelligence (AI) has emerged as a useful aid in numerous clinical applications for Computer Aided Diagnosis (CAD) and treatment decisions. AI is surpassing the performance of clinicians in many tasks owing to the rapid increase in the available data and computational power. In order to conform to the ethical principles of trustworthy AI, it is essential for the AI system to be transparent, robust, fair and should ensure accountability. The current deep learning based AI systems are referred to as black-boxes due to the vague understanding of the specifics concerning the decision making process.
Therefore, there is a need to explore explainability and interpretability of these AI models before they can be incorporated in the clinical workflow. Breast cancer is difficult to diagnose using mammograms due the inconspicuous nature of the breast masses and inherent signal-to-noise ratio. Hence, there are a large number of cases that are missed and misdiagnosed. Although there are a large number of proposed AI algorithms that claim to surpass human performance for breast mass classification and segmentation but their adoption in clinical practice is still limited due the black-box nature of these algorithms. We discuss various post-hoc interpretability methods for breast mass segmentation and classification in an effort to enhance the interpretability of deep learning-based automated breast mass classification and segmentation systems.
Presentation 2
Time: 12:30 - 13:00
Title: Prognostic radiomics models for locally advanced head and neck cancer patients.
Speaker: Simon A. Keek
Abstract: Patients that suffer from advanced head and neck squamous cell carcinoma (HNSCC) have a low average survival chance. Improving prognosis could improve this survival rate as it may help in clinical decision making. Radiomics features calculated from images of the tumour describe tumour size, shape, and pattern. These characteristics may be used to identify tumour (sub)types, which can subsequently be linked to patient survival. In two projects, we investigate the capability of radiomics to predict survival for advanced HNSCC, and compare and combine these with other known predictors of survival.
In the first project, we combine radiomics features with other clinical and biological biomarkers of survival of 809 HNSCC patients to make a prognosis before treatment. We compared the predicted prognosis with the actual outcome to see how well our model performs. Our model was able to make three distinct risk groups of low-, medium-, and high-survival patients with radiomics alone (CI of 0.67), but did not improve on a model with biological and clinical biomarkers (CI of 0.73). However, combining radiomics with these biomarkers result in a model that can very accurately predict OS in advanced HNSCC patients (CI of 0.79). With these findings, doctors may make a better judgement of treatment and follow-up per patient, which might improve clinical outcomes.
In the second project, a model based on radiomics features is combined and compared with a model based on genomics features for 142 patients. A model based on radiomics features was not able to significantly split the validation dataset in high- and low-survival patients with radiomics (CI of 0.68). Likewise, genomics was not able to significantly split validation dataset, with a lower predictive performance (CI of 0.55). Combining the two types of features did not result in a higher predictive performance (CI of 0.67). These negative results can be attributed to low patients counts, especially in the validation cohort (N = 34), and more research is needed to determine the (complementary) value of radiomics and genomics for HNSCC prognosis.
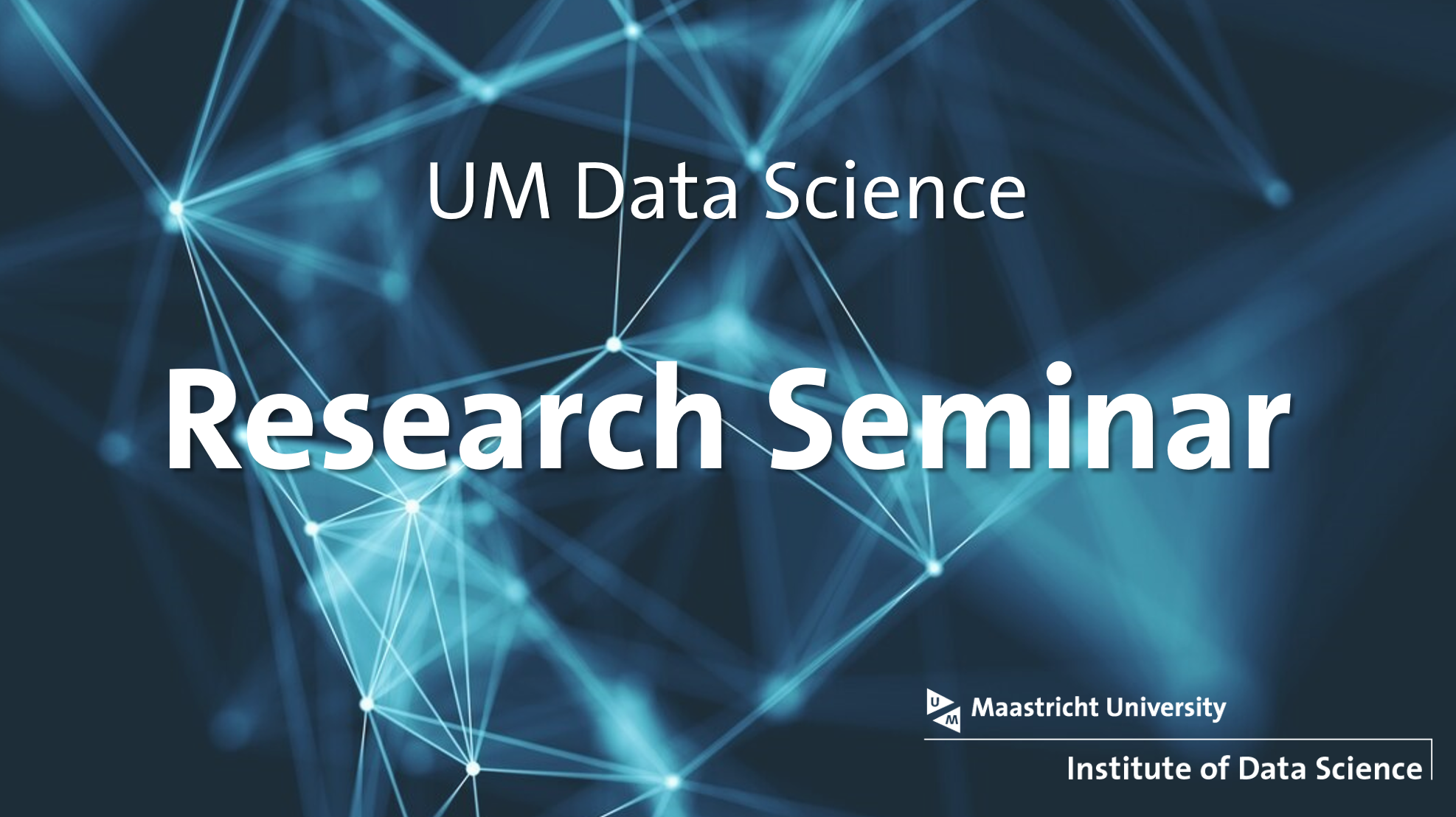
Online
This event is free and open to everyone. Please register before Thursday 19 August, 11:59 A.M.