Learning Analytics
Learning Analytics (LAs) are the measurement, collection, and analysis of educational-related data to understand and improve the learning and educational process in a given domain.
Learning Analytics refer to any information collected in the learning environment, such as:
- Feedback and evaluation forms
- Clicks, visualisations, and visualisation time of learning content/on the learning portal
- Test-based feedback
- Information collected by a learning managing system
Learning Analytics have a wide range of applications. Some examples include:
- Personalisation of education through adaptive learning (e.g., personalised homework assignments based on test results, personalised feedback)
- Early warning systems for students at risk
- Insights on results and performance aimed at curriculum improvement
- Insight into students’ satisfaction
- Insight into educators’ workload
- Understanding broader trends in education
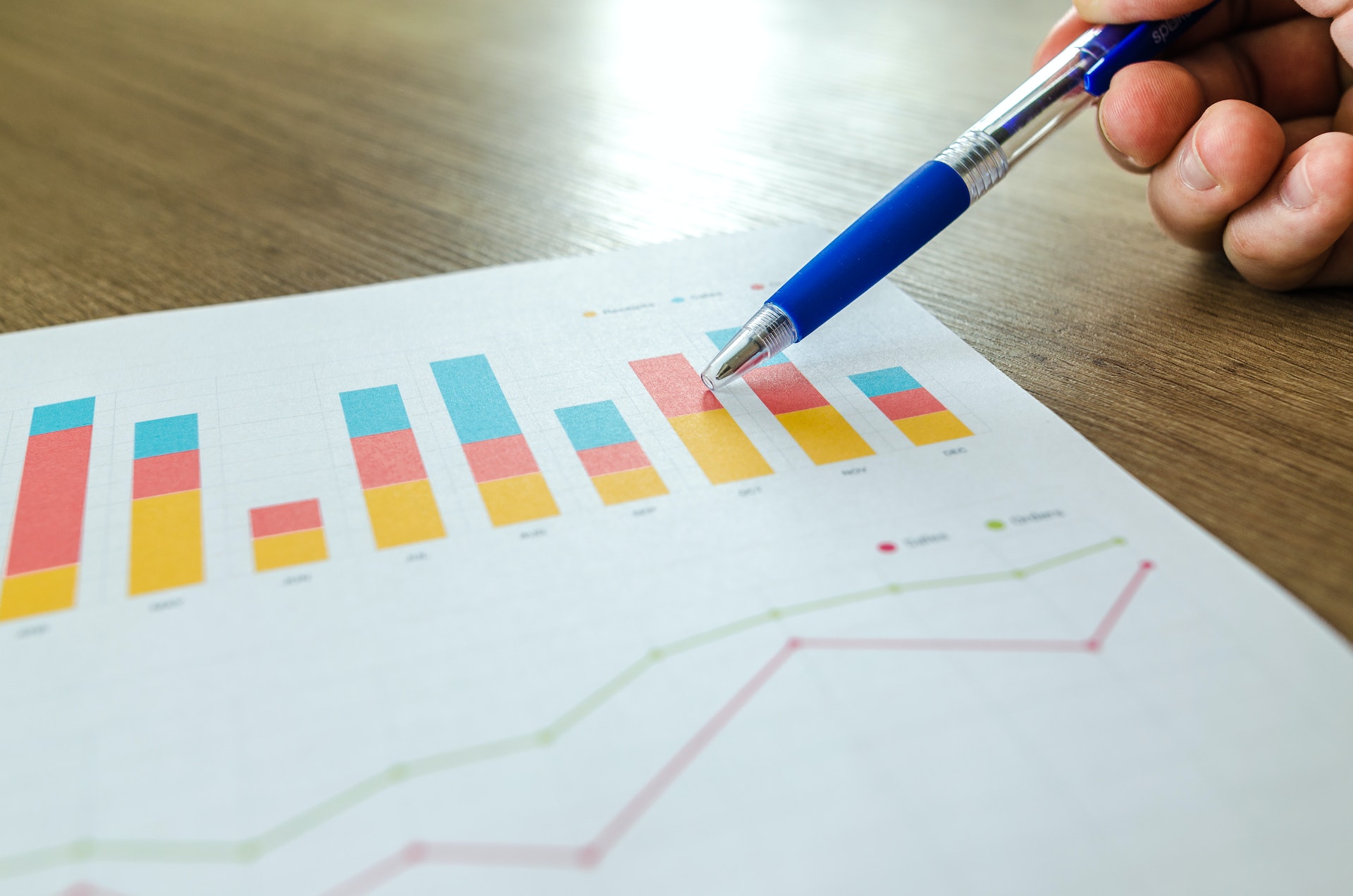
Learning Analytics have the potential to increase educational quality. However, they come with practical prerequisites and ethical and privacy-related risks.
Learning Analytics at Maastricht University: Lessons Learned
A previous project at Maastricht University concerning Learning Analytics generated the following lessons:
- LAs may help to provide student equity yet increase the risk of privacy infringements
- LAs seem to be particularly useful for courses with large (and rising) numbers of students
- LAs can foster incremental (and non-disruptive) quality change
- LAs avoid GDPR infringements with opt-in and opt-out declarations
- LAs combine learner development information based on Learning Analytics with suitable interventions, e.g., interpretation of the data, skills education or reflection with, e.g., peers, study advisors or mentors
- LAs allow for transparency, e.g., open data and algorithms
Mindful Use of Data & Adverse Effects on Learning
Impact on Self-directed Learning
The use of Learning Analytics can reduce the level of autonomy of students. LA-based predictions may interfere with students’ goal-setting, reflection and evaluation practices making the overall learning process less effective. Moreover, the predicted analysis might hinder students’ ability to choose their own learning path. At the same time, Learning Analytics can help teaching staff to better support students. The insights gained into student learning development can lead to improvement or adaptation of the educational offer for student batches or individual students.
Students & Data Predictions
Individuals may deal differently with LA-powered suggestions and feedback (in terms of interpretation skills) and have different ways to incorporate it into future learning behaviour. It is even shown that predictions may turn into “self-fulfilling prophecies”, meaning that if a student receives an ‘at-risk’-warning, they lose confidence and tend to drop out more quickly. There are limits to profiling or labelling students, even though it may also be unethical not to inform students when they are at risk. In any case, close follow-up by mentors, study advisors, or academic counsellors is important.
Teachers & Data Predictions
On the teacher's side, one should be hesitant to seek causation and draw conclusions based on data showing learner activity, engagement and study success. Depending on the assessment practices at the institution, students may, for example, be able to pass with limited class attendance, interaction, and Learning Management System (LMS) activity. High activity and engagement can be traits of the learning behaviour of both weak and strong students, and possible interventions will most likely differ. It is difficult to distinguish with limited data, and oversimplification is dangerous and may reduce individual learning preferences and circumstances to a manageable database of generic metrics.
Data quality and use
It remains a challenge to gather clean data that can be linked to one individual. Especially within the collaborative learning environment of Maastricht University, students can work together on assignments or use the same Learning Management System account (Canvas at UM). With such potential database “pollution”, the question remains whether data regarding LMS activity can be informative. To make informed evaluations, it is good practice to base decisions on a wide variety of data from different sources.
Data protection
Infringements with the General Data Protection Regulation (GDPR) and general privacy issues with learning analytics tend to surface with insecure anonymisation of data, re-usage and exploitation of data in different internal or external contexts, storage, security and the combination of datasets. The latter can lead to other correlations and conclusions and should always be prevented. Data ownership is tricky because the students remain owners of their data, yet monitoring and managing all extracted data is practically impossible. The institution is hence the main "administrator" of the collected data and is responsible for adhering to the privacy concerns mentioned above. Important questions regarding learning analytics and confidentiality that should be answered before implementation are, for example: What are legitimate goals for an institution to collect learner data? How can collected data be made insightful and transparent to the student?
Key Prerequisites for Using Learning Analytics
- For the optimal use of Learning Analytics, learning activities should be deployed within the institute's Learning Management System (Canvas at UM) as much as possible. Given Maastricht University's on-campus PBL environment it can not be expected (yet) that all learning activities take place within Canvas or other digital learning systems. This makes it difficult to collect the high amount and variety of data needed to obtain statistically significant insights into the learning process.
- Another aspect for optimal use of Learning Analytics is a clear visualisation of the information, e.g. through a dashboard, to gain insight and give meaning to results based on Learning Analytics.
Legal Considerations
When collecting, processing, and using data to make decisions, important legal pre-requisites for the use of Learning Analytics are:
- Openness
- Information
- Transparency
Moreover, we recommend to:
- Involve students (councils) in decisions to collect and make use of Learning Analytics
- Let any stakeholder know what data will be collected and for what purpose
- Ask students for informed consent for data extraction and use
- Clarify how long the learning data will be stored by the institution and respect student rights ‘to be forgotten’
- Offer students to opt out of data collection practices without being subjected to any negative (learning) consequences because of the opt-out
- Any stakeholder should be able to access and read the data collected by the institution.
References
- Sclater, N. (2017). Learning analytics explained. Taylor & Francis.
- Society for Learning Analytics Research (SoLAR). (2021, March 24). What is Learning Analytics?
- Van den Bogaard, M., et al. (2016). Learning Analytics in het onderwijs: een onderwijskundig perspectief. SURF.